Meritshot Tutorials
- Home
- »
- Understanding Data Types and Data Structure
Tableau Tutorial
-
Overview of TableauOverview of Tableau
-
Key Features and Benefits of TableauKey Features and Benefits of Tableau
-
Tableau Desktop vs. Tableau Online vs. Tableau ServerTableau Desktop vs. Tableau Online vs. Tableau Server
-
Navigating the Tableau InterfaceNavigating the Tableau Interface
-
Intro to Charts in TableauIntro to Charts in Tableau
-
Introduction to Calculated FieldsIntroduction to Calculated Fields
-
Common Calculations (e.g., Profit Margins, Growth Rates)Common Calculations (e.g., Profit Margins, Growth Rates)
-
Best Practices for Calculated FieldsBest Practices for Calculated Fields
-
Bar ChartBar Chart
-
Overview of Table CalculationsOverview of Table Calculations
-
Common Table Calculations (e.g., Running Total, Percent of Total)Common Table Calculations (e.g., Running Total, Percent of Total)
-
Customizing Table CalculationsCustomizing Table Calculations
-
Line ChartLine Chart
-
Aggregations in TableauAggregations in Tableau
-
Best Practices for AggregationBest Practices for Aggregation
-
Pie ChartPie Chart
-
Granularity in TableauGranularity in Tableau
-
Adjusting Granularity in Your VisualizationsAdjusting Granularity in Your Visualizations
-
Examples of Granularity in Different ScenariosExamples of Granularity in Different Scenarios
-
Scatter Plots in TableauScatter Plots in Tableau
-
Level of Detail (LOD) ExpressionsLevel of Detail (LOD) Expressions
-
Different Types of LOD Expressions (Fixed, Include, Exclude)Different Types of LOD Expressions (Fixed, Include, Exclude)
-
Practical Use Cases and ExamplesPractical Use Cases and Examples
-
HistogramsHistograms
-
Customizing Charts (Colors, Labels, Axes)Customizing Charts (Colors, Labels, Axes)
-
Introduction to Geographic DataIntroduction to Geographic Data
-
Creating and Refreshing Extracts in TableauCreating and Refreshing Extracts in Tableau
-
Benefits of Using Extracts vs. Live ConnectionsBenefits of Using Extracts vs. Live Connections
-
Creating Basic MapsCreating Basic Maps
-
Creating Interactive Filters (Dropdowns, Sliders)Creating Interactive Filters (Dropdowns, Sliders)
-
Using Filter Actions in DashboardsUsing Filter Actions in Dashboards
-
Customizing Maps (Layers, Annotations, Map Styles)Customizing Maps (Layers, Annotations, Map Styles)
-
Introduction to DashboardsIntroduction to Dashboards
-
Designing and Building DashboardsDesigning and Building Dashboards
-
Adding Interactivity (Actions, Filters)Adding Interactivity (Actions, Filters)
-
Using Map FiltersUsing Map Filters
-
Creating a Tableau StoryCreating a Tableau Story
-
Designing Storyboards for Effective CommunicationDesigning Storyboards for Effective Communication
-
Formatting in TableauFormatting in Tableau
-
Customizing Appearance (Colors, Borders, Fonts)Customizing Appearance (Colors, Borders, Fonts)
-
Best Practices for Dashboard FormattingBest Practices for Dashboard Formatting
-
Principles of Effective Data VisualizationPrinciples of Effective Data Visualization
-
Understanding Data Types and Data StructureUnderstanding Data Types and Data Structure
-
Choosing the Right Visualization for Your DataChoosing the Right Visualization for Your Data
-
Creating and Formatting ReportsCreating and Formatting Reports
-
Adding Filters and Parameters to ReportsAdding Filters and Parameters to Reports
-
Publishing and Sharing ReportsPublishing and Sharing Reports
Understanding Data Types and Data Structure
What Are Data Types?
Data types are fundamental concepts in data science and programming that define the kind of data that can be stored and manipulated within a system. Each data type determines the operations that can be performed on the data and how the data is stored in memory. Understanding data types is crucial for accurate data analysis, data manipulation, and for ensuring the efficiency of your code.
Here’s a breakdown of common data types:
- Numeric Data Types:
- Integer: Represents whole numbers without any decimal points. Examples: 1, 42, -7.
- Float/Double: Represents numbers with decimal points. Examples: 3.14, -0.001, 2.71828.
- Character Data Types:
- String: Represents sequences of characters. Examples: “Hello”, “Data Science”, “1234”.
- Char: Represents a single character. Examples: ‘a’, ‘X’, ‘7’.
- Boolean Data Type:
- Boolean: Represents binary values (True/False). Used for logical operations and comparisons.
- Date and Time Data Types:
- Date: Represents calendar dates. Examples: 2024-08-29, 1995-12-17.
- Time: Represents time of day. Examples: 14:30:00, 08:00:00.
- Datetime: Represents both date and time. Examples: 2024-08-29 14:30:00.
What Is Data Structure?
Data structures are ways to organize and store data so that it can be accessed and modified efficiently. Different data structures are suited for different types of operations and problems. Understanding data structures is essential for designing effective algorithms and managing data in programming and data science.
Here’s a look at common data structures:
- Arrays:
- Definition: A collection of elements identified by index or key. All elements are of the same data type.
- Use Case: Storing and accessing sequential data quickly. Example: A list of temperatures for each day of the month.
- Lists:
- Definition: An ordered collection of elements that can be of different data types. Lists are dynamic and can grow or shrink in size.
- Use Case: Storing a collection of items where the size is not fixed. Example: A list of student names in a class.
- Dictionaries:
- Definition: A collection of key-value pairs where each key is unique. Allows for fast retrieval based on keys.
- Use Case: Storing data with a unique identifier. Example: A dictionary of employee IDs and names.
- Sets:
- Definition: An unordered collection of unique elements. No duplicate values are allowed.
- Use Case: Removing duplicates and performing set operations like union and intersection. Example: A set of unique tags for blog posts.
- Tuples:
- Definition: An ordered collection of elements that can be of different data types. Tuples are immutable, meaning they cannot be modified after creation.
- Use Case: Grouping related data together that should not be changed. Example: Coordinates (latitude, longitude).
Examples of Data Types and Structures in Practice
- Numeric Data Types:
- Example: Analyzing the sales figures (floats) over a month (integers representing days).
- String Data Types:
- Example: Categorizing customer reviews by sentiment (positive, neutral, negative).
- Boolean Data Type:
- Example: Filtering out users who have purchased a product (True) vs. those who haven’t (False).
- Date and Time Data Types:
- Example: Tracking user logins over a week or analyzing time series data.
- Arrays:
- Example: Storing weekly temperatures for easy access and calculation.
- Lists:
- Example: Creating a list of project tasks with various attributes.
- Dictionaries:
- Example: Mapping product IDs to product names in an inventory system.
- Sets:
- Example: Identifying unique customer IDs who made a purchase.
- Tuples:
- Example: Storing data related to a user’s profile, like (username, email, date of birth).
Frequently Asked Questions
Q1: Why is it important to choose the correct data type?
A1: Choosing the correct data type ensures that operations on the data are efficient and that the data is stored correctly. It helps avoid errors and improves performance.
Q2: What happens if you use the wrong data type?
A2: Using the wrong data type can lead to data corruption, errors in calculations, inefficient memory use, and unexpected results.
Q3: How do you decide which data structure to use?
A3: The choice of data structure depends on the type of operations you need to perform, such as searching, sorting, or accessing data. Consider the nature of your data and the operations you’ll perform.
Q4: Can you change the data type of a variable once it is set?
A4: Yes, many programming languages allow you to convert between data types using typecasting functions. For example, converting a string to an integer or vice versa.
Q5: How do data structures affect the efficiency of algorithms?
A5: Different data structures offer different performance benefits for various operations. Choosing the right data structure can significantly impact the efficiency and speed of algorithms.
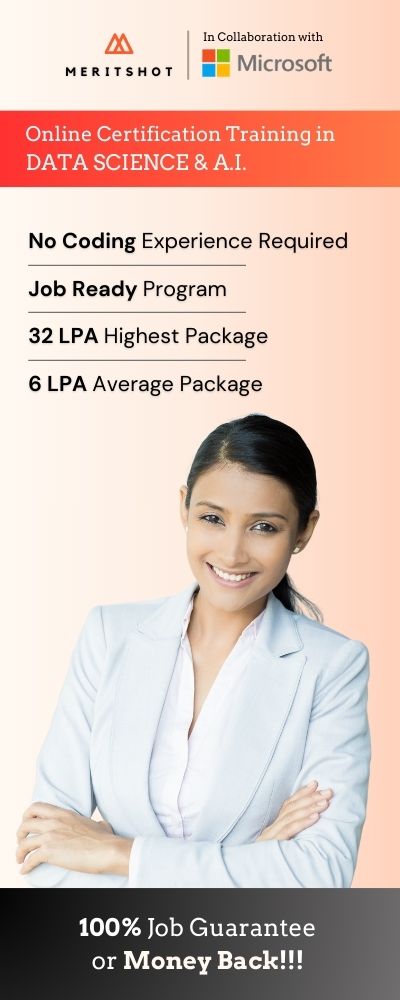